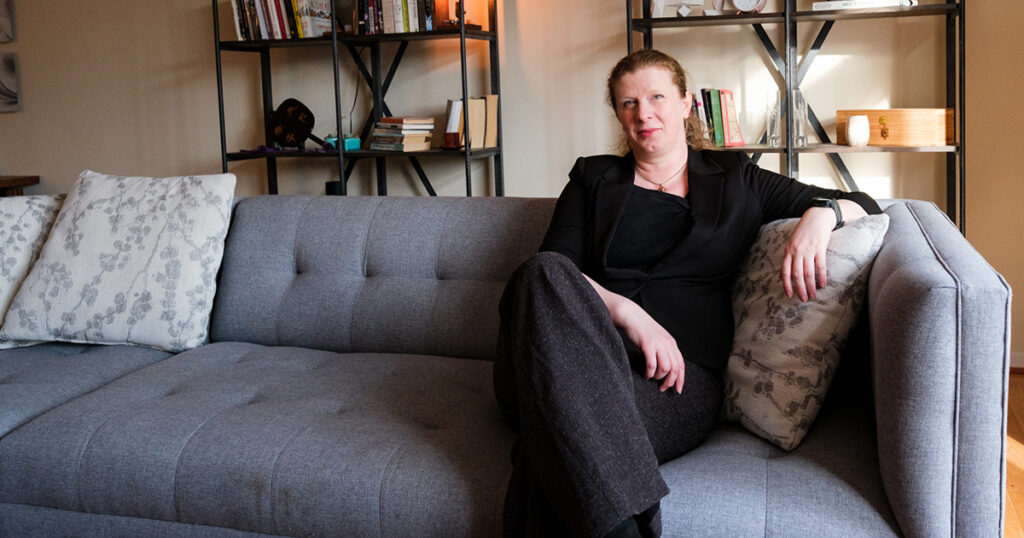
The use of AI tools in healthcare is accelerating, with increasing confidence in the results they produce and deliver.
RAMP, one such tool already in operation at UVA Health, focuses on delivering actionable, verifiable, and explainable machine learning, and integrating it as a decision support tool into clinical workflows to improve insights into patient health trends, facilitate faster delivery of needed care, and improve patient well-being. Results.
AI-based predictive analytics models use complex real-time and historical patient data to provide healthcare professionals with actionable insights, alerting care teams if a patient needs immediate attention.
Valentina Baljac is a senior data scientist at UVA Health. She holds a PhD in Information Science and Technology, and Applied Machine Learning. RAMP was created by UVA Health and is used today.
Baljak and two colleagues will discuss AI, RAMP, and more at HIMSS25 in March in Las Vegas in their session titled “Real-Time Analytics Monitoring Platform: Usable AI in Action.” We spoke with Baljak to understand what she and her colleagues will talk about in the session and what HIMSS25 attendees can hope to take away from their talk.
S. What is the primary topic you will cover in your session and why is it relevant to healthcare and health IT today?
A. With the recent emergence of generative AI models, this topic is receiving greater attention in the healthcare field. In this work, we focus on real-time clinical decision support tools. Artificial intelligence is not a new term.
At UVA Health, we’ve been developing real-time predictive systems for several years now, and one of the biggest lessons we’ve learned is that the form AI should take is the form that best meets your needs. Doctors won’t be left behind with tools they can’t interpret. Building trust in our models and tools has meant close collaboration at every step, from day one.
We want to provide a detailed outline of how to build a system that works in your environment, and to raise awareness of the importance of transparency, accountability, and explainability of your models. This is especially important in the medical field, with real-time predictions that can have a significant impact on patient outcomes.
Q: You will be focusing heavily on artificial intelligence. How is it used in healthcare within the context of your session focus?
A. A key aspect of RAMP is the collection of real-time data from the electronic health record and other data sources. The ability to write results back to patient records in the electronic health record and alert care teams in real time makes RAMP a critical tool in the clinical setting.
The technologies used here are fairly well established and are all open source. Python provides a solid foundation for machine learning development, back-end communication, and data processing. Connections to various data sources are established using FiHR, REST API and custom HL7. The website is built using Angular.
As our latest major expansion, we are building a new predictive model on top of our largest real-time data stream, built using Kafka to collect all vital and ECG waveforms from bedside monitors.
Q: Attendees will come to your session seeking to bring knowledge home. What takeaways can they expect?
A. Artificial intelligence is an essential part of modern healthcare, and takes different forms as needed. Choosing the right approach to AI is crucial, given the high stakes.
If you have in-house expertise and resources, developing a custom AI system is a powerful alternative to vendor-supplied black box systems.
Valentina Baljak’s session, “Real-Time Analytics Monitoring Platform: Usable AI at Work,” is scheduled for Tuesday, March 4, at 12:45 p.m. in HIMSS25 in Las Vegas.